Morphology of the cosmic web
A natural application of Bayesian large-scale structure inference with BORG is a high-fidelity description of the complex web-like patterns in cosmic structure.
Dark matter voids
An interesting aspect is voids in the dark matter distribution. In 2014, we produced and analysed constrained catalogues of dark matter voids in the Sloan volume, probing a deeper void hierarchy and than the distribution of galaxies and alleviating the issues due to sparsity and bias (Leclercq et al. 2015a). The catalogues have been made publicly available here and on cosmicvoids.net.
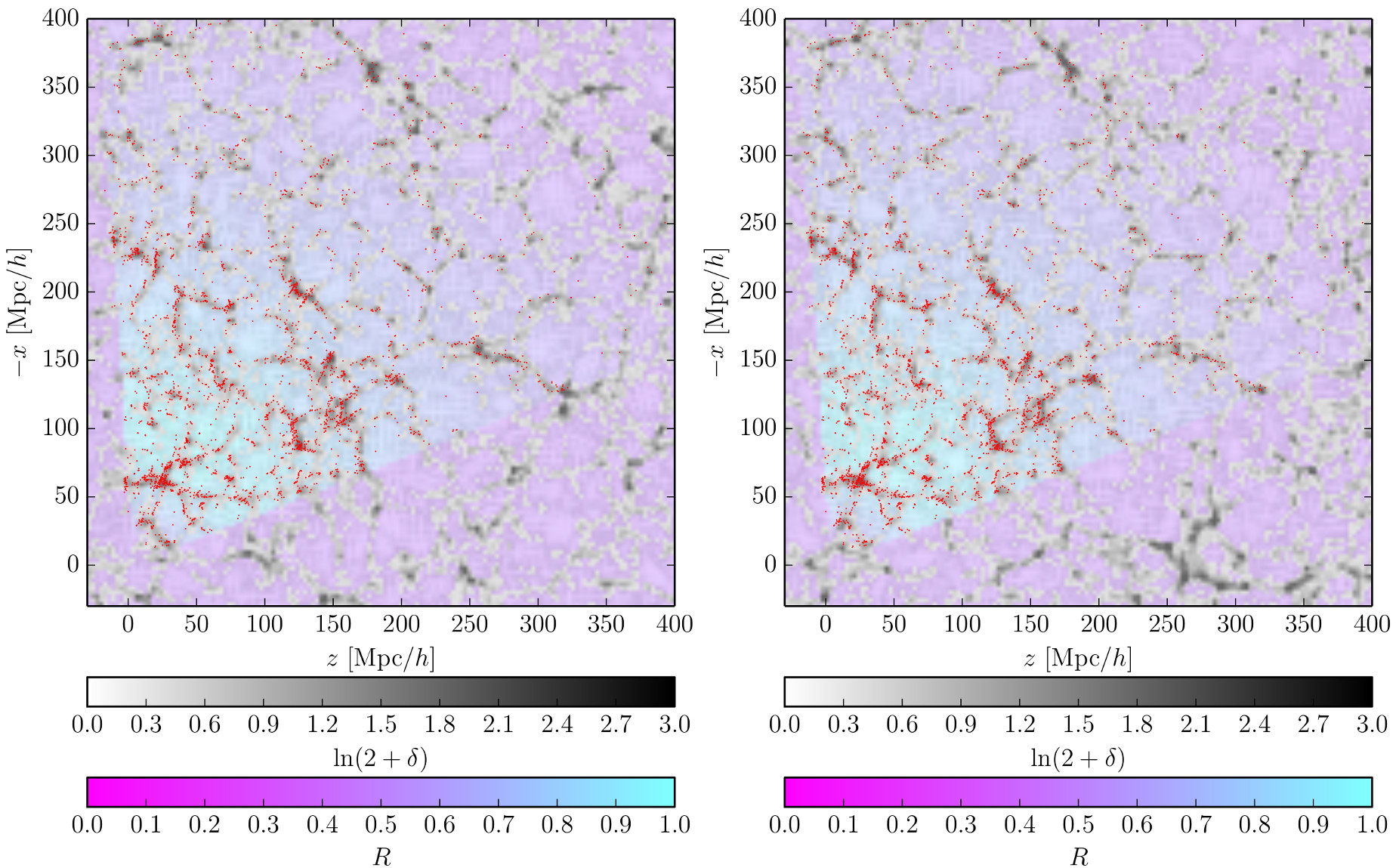
The dynamic cosmic web
More generally, BORG infers a wealth of cosmological information that can be used to classify the large-scale structure. Following the dynamic cosmic web classification procedure proposed by Hahn et al. (2007), we used the tidal field to classify the large-scale structure into four distinct web-types (voids, sheets, filaments, and clusters) and quantify corresponding uncertainties (Leclercq et al. 2015b).
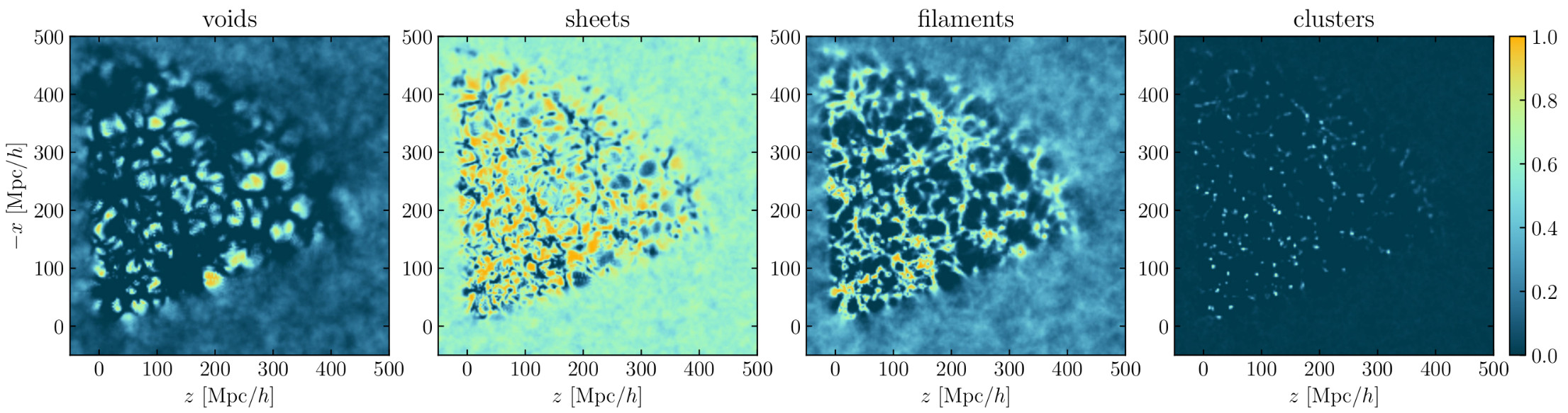
Within the same project, we also introduced a new cosmic web classifier, LICH, to identify structures while taking into account the existence of both potential and vortical flows. Using it as well a set of phase-space techniques resulted in the first phase-space analysis of the nearby Universe as probed by the SDSS (Leclercq et al. 2017), which was the object of a press release.
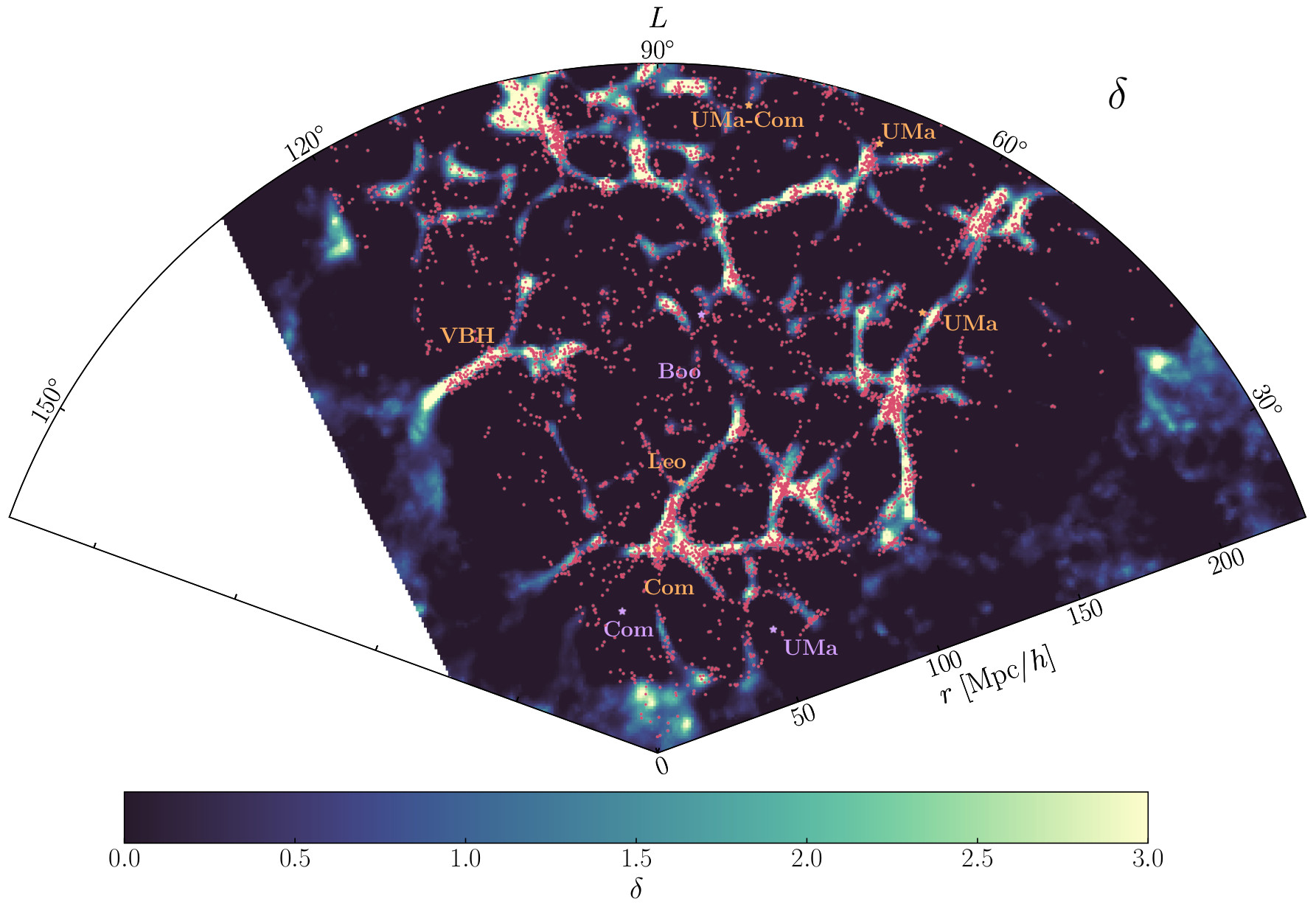
Cosmic web analysis using decision theory and information theory
With Jens Jasche and Benjamin Wandelt, I proposed to use Bayesian decision theory for segmenting the cosmic web into different structure types, on the basis of their (previously inferred) probabilities (Leclercq et al. 2015c). Surprisingly, our approach is analogous to the analysis of games of chance where the gambler only plays if a positive expected net gain can be achieved based on some degree of privileged information.
In 2016, we extended the decision problem to the space of cosmic web classifiers (Leclercq et al. 2016). Our framework, based on information theory, accounts for the design aims of different classes of possible applications: parameter inference, model selection, and prediction of new observations. As an illustration, we assessed the relative performance of different classifiers (the T-web, DIVA, and ORIGAMI) for respectively: analysing the morphology of the cosmic web, discriminating dark energy models, and predicting galaxy colours.
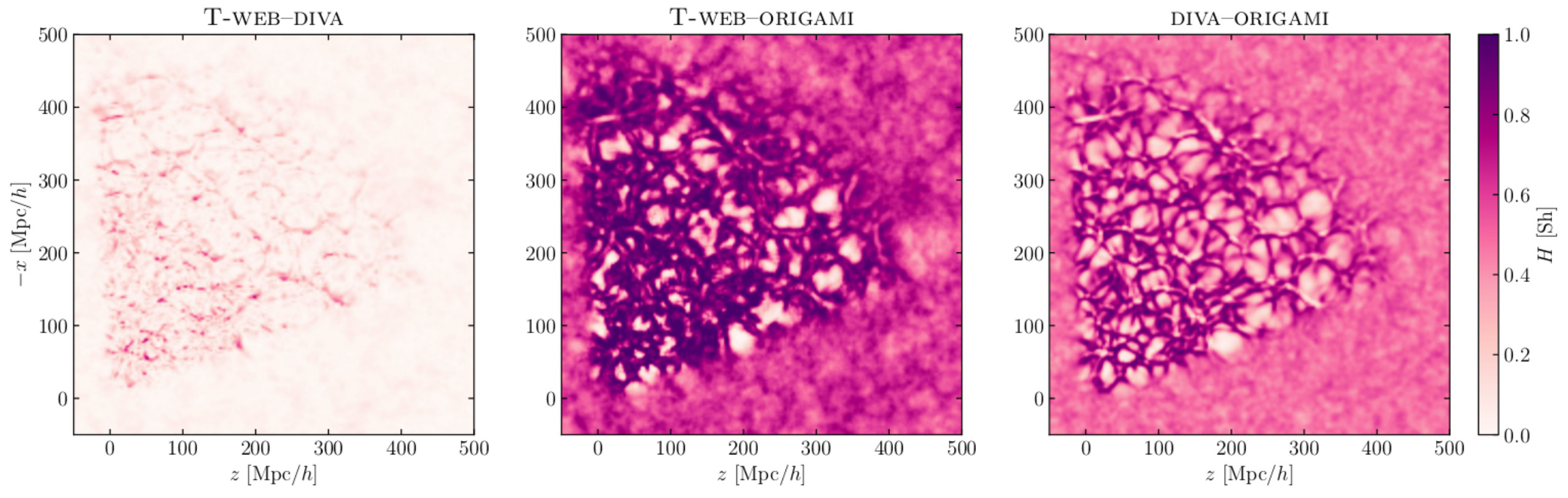