Bayesian large-scale structure inference
Much of my PhD work relied on the full-scale Bayesian code BORG (Bayesian Origin Reconstruction from Galaxies, Jasche & Wandelt 2013, Jasche, Leclercq & Wandelt 2015, Lavaux & Jasche 2016), an algorithm developed for large-scale structure inference in the linear and mildly non-linear regime of cosmic structure formation, with accurate capability of uncertainty quantification. BORG paves the path toward high precision chrono-cosmography, the subject of simultaneously studying the dynamics and the morphology of the inhomogeneous Universe.
Samples of the posterior distribution are obtained via an efficient implementation of the Hamiltonian Markov Chain Monte Carlo method (Duane et al. 1987). The algorithm self-consistently accounts for observational uncertainty such as noise, survey geometry, selection effects and luminosity-dependent galaxy biases (Jasche & Wandelt 2013). It accurately accounts for all non-linearities and non-Gaussianities involved in the inference process and achieves high statistical efficiency, even in low signal-to-noise regimes.
Each inferred sample (each frame in the video below) is a “possible version of the truth” for the formation history of the large-scale structure, in the form of a full physical realisation of dark matter particles. The variation between samples accounts for joint and correlated uncertainties. In particular, it quantifies complex information propagation, translating uncertainties from observations to inferred initial conditions.
BORG at work. Each frame of the video shows slices through one sample of inferred density fields. Left panel: initial conditions. Middle panel: final conditions. Right panel: galaxy number count in the SDSS DR7.
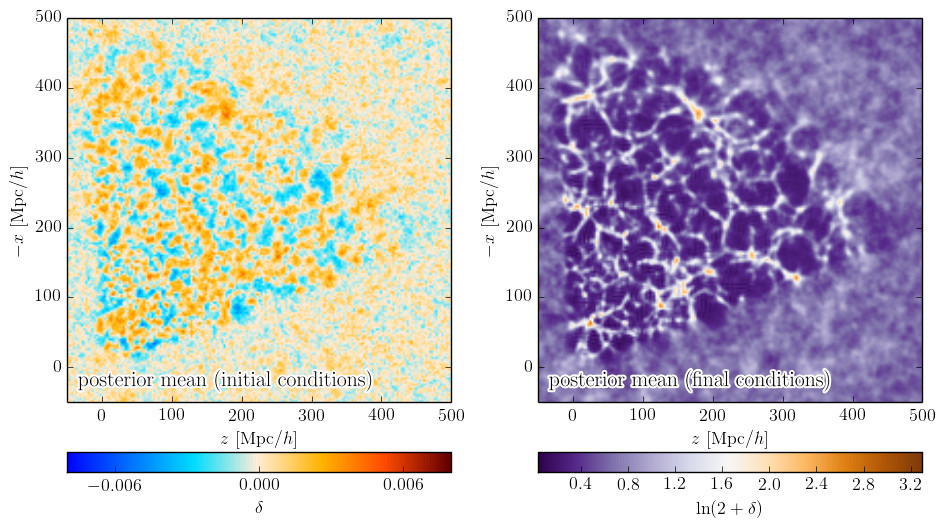